The Role of AI in Cybersecurity: Opportunities and Risks
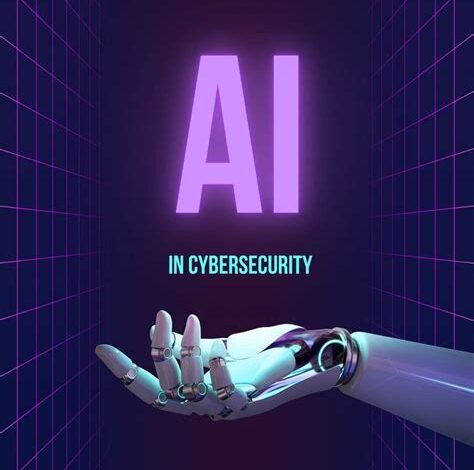
This blog post explores the role of Artificial Intelligence in modern cybersecurity, highlighting both its potential and inherent risks. It begins with an overview of contemporary cybersecurity threats, then delves into how AI enhances threat detection and prevention through advanced algorithms and automation. However, the article also acknowledges AI’s ‘double-edged sword’ nature, examining vulnerabilities that malicious actors can exploit. Finally, it provides best practices for mitigating these risks and discusses future directions in AI-driven cybersecurity to ensure a safer digital landscape. Understanding these aspects of AI is crucial for navigating the evolving threat landscape.
Understanding Cybersecurity Threats: An Introduction
In today’s digital landscape, understanding cybersecurity threats is more critical than ever. As businesses and individuals increasingly rely on technology, they become more vulnerable to a wide array of malicious activities. Recognizing the common types of threats and their potential impact is the first step in building a robust defense strategy. This section provides an overview of the prevalent cybersecurity threats that organizations and individuals face daily, setting the stage for exploring the role of AI in mitigating these risks.
Cybersecurity threats come in many forms, each designed to exploit vulnerabilities in systems and networks. From simple phishing scams to sophisticated ransomware attacks, the goal is often the same: to gain unauthorized access to sensitive information, disrupt operations, or extort money. Staying informed about these threats and their evolving nature is essential for maintaining a strong security posture. Awareness helps in implementing proactive measures and responding effectively when an incident occurs.
Common Types of Cybersecurity Threats:
- Malware: Malicious software designed to disrupt, damage, or gain unauthorized access to a computer system.
- Phishing: Deceptive attempts to acquire sensitive information, such as usernames, passwords, and credit card details, by disguising as a trustworthy entity.
- Ransomware: A type of malware that encrypts a victim’s files, demanding a ransom to restore access.
- Denial-of-Service (DoS) Attacks: Overwhelming a system with traffic to make it unavailable to legitimate users.
- SQL Injection: Exploiting vulnerabilities in database-driven applications to inject malicious SQL statements.
- Cross-Site Scripting (XSS): Injecting malicious scripts into trusted websites to target users.
To further illustrate the diverse landscape of cybersecurity threats, consider the following table, which categorizes different threat types by their primary objectives and potential impacts. Understanding these distinctions is vital for developing targeted security strategies and allocating resources effectively. Being proactive and informed is the best defense against these ever-evolving threats.
Threat Type | Primary Objective | Potential Impact |
---|---|---|
Malware | Infect systems, steal data | Data loss, system corruption, financial loss |
Phishing | Obtain sensitive information | Identity theft, financial fraud, data breaches |
Ransomware | Extort money | Business disruption, data loss, financial strain |
DoS Attacks | Disrupt services | Service unavailability, reputational damage, loss of productivity |
In conclusion, recognizing and understanding cybersecurity threats is paramount for protecting digital assets and maintaining operational integrity. As threats become more sophisticated, organizations must adopt a proactive and adaptive approach to cybersecurity. This includes staying informed about the latest threats, implementing robust security measures, and leveraging advanced technologies like AI to enhance threat detection and prevention. As quote>the cybersecurity landscape continues to evolve, a comprehensive understanding of these threats will be the cornerstone of effective defense strategies.
The Role of AI in Threat Detection and Prevention
Artificial intelligence is revolutionizing cybersecurity, offering powerful tools for both threat detection and prevention. As cyber threats become more sophisticated and frequent, traditional security measures often struggle to keep pace. AI’s ability to analyze vast amounts of data, identify patterns, and automate responses provides a critical advantage in defending against these evolving threats. In this section, we’ll explore how AI is transforming the landscape of cybersecurity, enhancing our ability to detect anomalies, automate threat intelligence, and predict potential security breaches. The integration of AI is not merely an upgrade; it represents a paradigm shift in how organizations approach and manage their security postures.
One of the key advantages of AI in cybersecurity is its capacity to process and analyze data at a scale and speed that far exceeds human capabilities. This allows for the identification of subtle anomalies and patterns that might otherwise go unnoticed. Furthermore, AI-driven systems can continuously learn and adapt, improving their accuracy and effectiveness over time. Below is a table illustrating how AI compares to traditional methods in key areas of threat detection:
Feature | Traditional Methods | AI-Powered Methods |
---|---|---|
Data Analysis Speed | Slow, manual analysis | Rapid, automated analysis |
Pattern Recognition | Limited to known patterns | Identifies novel and complex patterns |
Adaptability | Requires manual updates | Continuously learns and adapts |
Scalability | Difficult to scale | Highly scalable |
Here’s a structured overview of how AI enhances threat detection, offering a layered and dynamic defense mechanism. Understanding these enhancements is crucial for leveraging AI effectively in cybersecurity strategies.
- Real-Time Analysis: AI provides instant analysis of network traffic and system behavior.
- Behavioral Biometrics: AI can identify unusual user activities by learning normal behavior patterns.
- Automated Response: AI automates actions to isolate and eliminate threats.
- Predictive Analysis: AI predicts future threats by examining historical data and trends.
- Vulnerability Scanning: AI autonomously scans systems to find potential vulnerabilities.
AI-Powered Anomaly Detection
AI-powered anomaly detection is a critical component of modern cybersecurity. By continuously monitoring network traffic, system logs, and user behavior, AI algorithms can establish a baseline of normal activity. Any deviation from this baseline is flagged as a potential anomaly, which can then be investigated further. This proactive approach allows security teams to identify and respond to threats before they can cause significant damage. For example, if an employee suddenly starts accessing files they don’t typically access, the AI system can flag this as suspicious behavior.
Automated Threat Intelligence
Automated threat intelligence leverages AI to gather, analyze, and disseminate information about potential threats. AI algorithms can scour the internet, dark web, and other sources to identify emerging threats, vulnerabilities, and attack patterns. This information is then used to update security systems and inform defensive strategies. By automating this process, organizations can stay ahead of the curve and proactively defend against the latest threats. This allows cybersecurity professionals to stay one step ahead, anticipating and neutralizing threats before they materialize.
Predictive Security Analytics
Predictive security analytics uses AI to analyze historical data and identify patterns that can be used to predict future security breaches. By identifying potential vulnerabilities and predicting where attacks are likely to occur, organizations can take proactive steps to strengthen their defenses. This might involve patching vulnerabilities, implementing stricter access controls, or increasing monitoring in high-risk areas. Predictive analytics is a powerful tool for reducing the risk of cyberattacks and minimizing the impact of breaches when they do occur. Furthermore, AI can help organizations prioritize their security efforts, focusing on the areas that pose the greatest risk.
The power of AI in cybersecurity lies in its ability to learn, adapt, and automate, providing a dynamic defense against an ever-changing threat landscape.Okay, I will create the AI’s Double-Edged Sword: Risks and Vulnerabilities section for your blog post, following all your instructions. html
AI’s Double-Edged Sword: Risks and Vulnerabilities
While AI offers significant advantages in cybersecurity, it also presents new risks and vulnerabilities that must be addressed. The very technologies that empower threat detection and prevention can also be exploited by malicious actors. Understanding these potential pitfalls is crucial for developing a comprehensive cybersecurity strategy. The role of AI in cybersecurity is not without its challenges, and a proactive approach is necessary to mitigate the dangers.
- Data Poisoning: Attackers can manipulate the training data used to develop AI models, causing them to make incorrect predictions or classifications.
- Adversarial Attacks: Carefully crafted inputs can fool AI systems, leading them to misidentify threats or bypass security measures.
- Model Extraction: Malicious actors can reverse engineer AI models to steal sensitive information or create copies for their own use.
- AI-Powered Social Engineering: AI can be used to create more convincing and personalized phishing attacks.
- Automated Hacking: AI can automate the process of finding and exploiting vulnerabilities in systems.
- Bias Amplification: AI systems can perpetuate and amplify existing biases in data, leading to unfair or discriminatory outcomes.
One of the primary concerns is the potential for AI to be used to create more sophisticated and evasive malware. Cybercriminals can leverage AI to automate the process of finding vulnerabilities, crafting exploits, and evading detection. This can lead to a significant increase in the volume and complexity of cyberattacks, making it more difficult for organizations to defend themselves.
Risk | Description | Mitigation Strategy |
---|---|---|
Data Poisoning | Manipulation of training data to compromise AI model accuracy. | Data validation, anomaly detection, and robust training techniques. |
Adversarial Attacks | Crafting specific inputs to fool AI systems. | Adversarial training, input validation, and model hardening. |
Model Extraction | Reverse engineering AI models to steal sensitive information. | Model obfuscation, access controls, and data encryption. |
AI-Powered Social Engineering | Using AI to craft more convincing phishing attacks. | User awareness training, multi-factor authentication, and email security solutions. |
Furthermore, the reliance on AI in cybersecurity can create a single point of failure. If an AI system is compromised or malfunctions, it can have a cascading effect, leaving organizations vulnerable to attack. It’s essential to implement redundancy and fail-safe mechanisms to ensure that critical security functions are not entirely dependent on AI. Continuous monitoring and auditing of AI systems are also necessary to detect and respond to any anomalies or vulnerabilities.
In conclusion, while AI offers tremendous potential for enhancing cybersecurity, it is crucial to acknowledge and address the associated risks and vulnerabilities. A proactive and multi-faceted approach is needed to mitigate these threats and ensure that AI is used responsibly and ethically in the fight against cybercrime. The future of cybersecurity depends on our ability to harness the power of AI while safeguarding against its potential misuse.
Here’s the content section for your article:
Mitigating AI Risks: Best Practices and Future Directions
As AI continues to revolutionize cybersecurity, understanding and mitigating its inherent risks becomes paramount. Organizations must proactively adopt strategies to safeguard against potential vulnerabilities exploited by malicious actors. A multi-faceted approach, encompassing robust security protocols, continuous monitoring, and ethical AI development, is crucial for ensuring the safe and responsible integration of AI in cybersecurity. Addressing these challenges head-on will pave the way for a future where AI’s power is harnessed for good, fortifying our digital defenses without creating new weaknesses.
Risk Area | Mitigation Strategy | Implementation Tools |
---|---|---|
Data Poisoning | Implement data validation and sanitization techniques | Data integrity monitoring systems, anomaly detection algorithms |
Adversarial Attacks | Employ adversarial training and defensive distillation | Robust optimization libraries, certified defense mechanisms |
Model Theft | Utilize model watermarking and access control mechanisms | Digital watermarking tools, role-based access control systems |
Bias Amplification | Ensure diverse training datasets and fairness-aware algorithms | Bias detection toolkits, fairness metrics dashboards |
Effective risk mitigation also requires a commitment to ongoing education and training. Cybersecurity professionals need to stay abreast of the latest AI threats and defensive techniques. Furthermore, fostering collaboration between AI developers, cybersecurity experts, and policymakers is essential for establishing industry-wide standards and best practices. By working together, we can create a more secure and resilient AI ecosystem, minimizing the potential for misuse and maximizing its benefits.
Actionable Steps for Mitigating AI Risks:
- Implement rigorous data validation and sanitization processes to prevent data poisoning attacks.
- Employ adversarial training techniques to enhance the robustness of AI models against malicious inputs.
- Utilize model watermarking and access control mechanisms to protect against model theft and unauthorized use.
- Prioritize the use of diverse and representative datasets to mitigate bias amplification in AI systems.
- Establish clear ethical guidelines and governance frameworks for the development and deployment of AI in cybersecurity.
- Continuously monitor AI systems for anomalies and vulnerabilities, and promptly address any identified issues.
Looking ahead, the future of AI risk mitigation lies in the development of more sophisticated and adaptive security solutions. This includes exploring techniques such as federated learning, which allows AI models to be trained on decentralized data sources without compromising privacy, and explainable AI (XAI), which enhances transparency and trust in AI decision-making. Ultimately, the role of proactive risk management is crucial in harnessing the full potential of AI in cybersecurity while safeguarding against its inherent vulnerabilities.